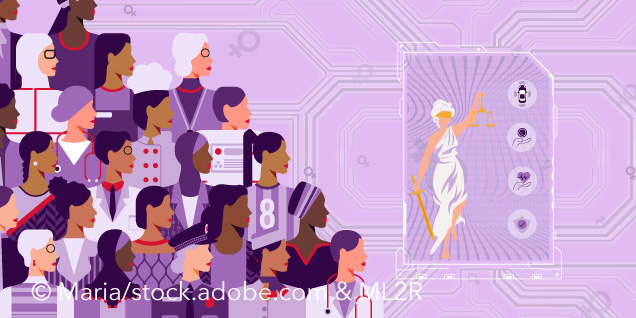
In this post, Dr. Maximilian Poretschkin, a scientist and team leader for AI assurance and certification at Fraunhofer IAIS, provides a concise overview of the topic of trustworthiness in Artificial Intelligence and explains why it is crucial to establish standardized testing procedures for AI systems. He not only answers the question “What needs to be tested?” but also delves into why ensuring and demonstrating the trustworthiness of an AI system is essential.
Artificial Intelligence (AI) is entering more and more areas of our lives, increasingly taking on responsible tasks, such as in highly automated driving assistance systems, applications for medical image analysis, process automation in insurance, or creditworthiness assessments in banks. The acceptance of AI in sensitive areas will only be sufficient if end-users and stakeholders can trust that such AI applications function correctly and have been developed to high-quality standards. Independent evaluations of AI applications (by an impartial party) have proven to be a reliable way to build trust. The result of such an evaluation can be a report, quality seal, or certificate that visibly demonstrates the adherence to quality standards of AI systems to third parties. The current draft legislation by the European Commission for regulating AI systems also calls for the assessment of high-risk AI applications.
What needs to be tested?
To realize such AI assessments, the first question is what requirements an AI application should be tested against. These requirements are summarized by the concept of AI trustworthiness, which includes various dimensions as presented below:
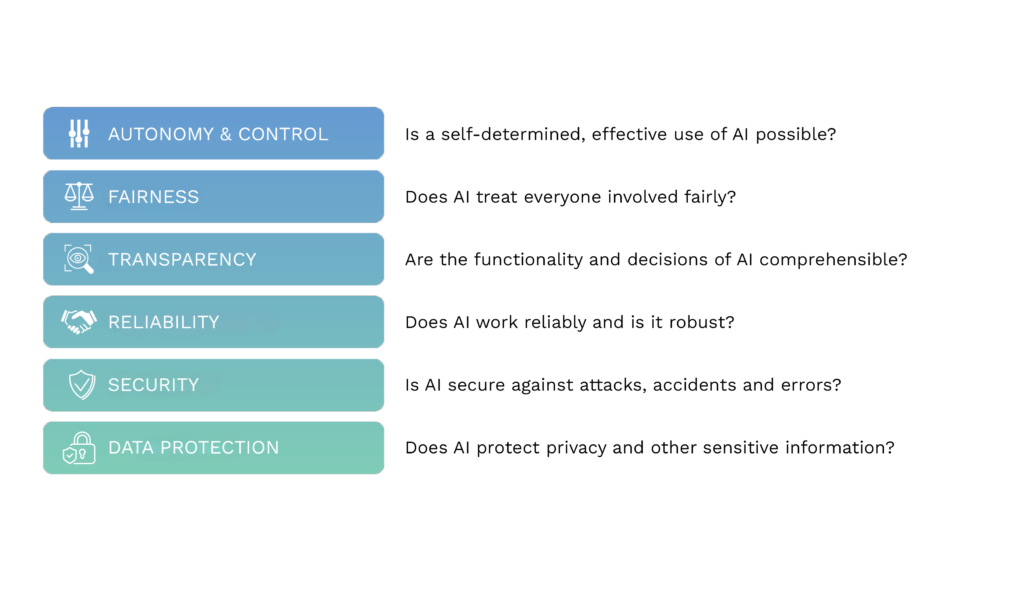
AI trustworthiness encompasses multiple dimensions, including control, transparency, and security. The “Ethics Guidelines for trustworthy AI” by the High-Level Expert Group on Artificial Intelligence (HLEG AI) of the European Commission provide an initial draft of guidelines for trustworthy AI.
In individual cases, not all requirements are equally important for all AI applications, but their significance depends on the specific context of the AI application. For example, an application for predictive maintenance of machines is unlikely to unjustifiably discriminate against users of the application. In this case, the “fairness” dimension is of lower importance. However, for an AI application assisting in creditworthiness assessments, the “fairness” risk is crucial and should be thoroughly examined in the assessment.
Why is the development of AI assessments a matter for research?
AI applications are fundamentally based on software, which is implemented by Artificial Intelligence, such as an ML model. While there are established procedures to systematically test and verify software, these fall short in the case of AI. One significant reason is that many AI applications, especially ML models, are data-driven and learn their internal logic from adapting certain parameters to large datasets. This poses several challenges for research, and two prominent issues are briefly outlined below:
Validation of AI applications: “Conventional software” is typically modularly structured, allowing it to be systematically tested by breaking it down into small units, testing each unit, and aggregating the test results of individual units to make a statement about the entire software. This is not easily achievable in the case of AI: a neural network usually cannot be broken down into individual components for testing. It can only be tested as a whole, making the testing process very complex. Particularly for networks that must handle a vast amount of possible input data (in an open-world context where the potential input space is virtually unlimited), determining the test coverage is very challenging. There are various approaches to address this: for specific network architectures, activation functions, and specifications of the input space, it is possible to make mathematically provable statements about limitations of the space of possible outputs. However, for many AI applications, this approach is not yet practical, so research focuses on developing approaches for systematic testing.
On-the-job learning: AI applications based on ML models can – at least in principle – continue to learn during productive use. Additionally, an AI application can change its behavior even after the learning process is complete, namely when its operational environment changes and the input data to be processed are no longer structurally identical to the training datasets. This poses a challenge for traditional testing procedures because a test result at a fixed point in time may lose its validity if the subject of the test is significant changed afterwards. Therefore, the development of procedures that enable continuous “real time” testing of AI systems is necessary.
The progress in addressing these issues must be continuously incorporated into standardization processes, as standards and norms serve as reference points against which assessments take place. There is still significant work to be done in this regard, as highlighted by the DIN and DKE Standardization Roadmap for AI.
What are the possible solutions?
Examining testing procedures from adjacent domains, such as IT security, reveals roughly two prominent approaches to realize assessments: process and product testing. The former type of testing addresses the processes by which AI applications are developed and operated, based on the assumption that good processes also lead to good AI applications. Moreover, many AI applications cannot operate without accompanying processes, which also emphasizes the importance of such assessments. The second type of testing aims to validate the functionalities of a specific AI application or, through structured risk analysis, demonstrate that significant risks regarding trustworthiness are adequately mitigated. In this context, Fraunhofer IAIS has published the first AI testing catalog, the “Guideline for Designing Trustworthy AI”, which summarizes the state of the art on the research questions outlined above and has been used in initial AI assessments.