Trustworthy Artificial Intelligence
Trustworthy AI builds on robust, verifiable procedures and forms the basis for certifying AI applications.
Trustworthiness has many facets and concerns, apart from computer science, diverse subjects, ranging from psychology and philosophy to economy and law. Consequently, the scientists from the Lamarr Institute who are working on trustworthy applications of Artificial Intelligence are members of an interdisciplinary team. For their research, they consider the overall pipeline of data gathering, storing, accessing, sampling, preprocessing, model selection, modeling, adapting, and applying the model to a process with a certain outcome and impact.
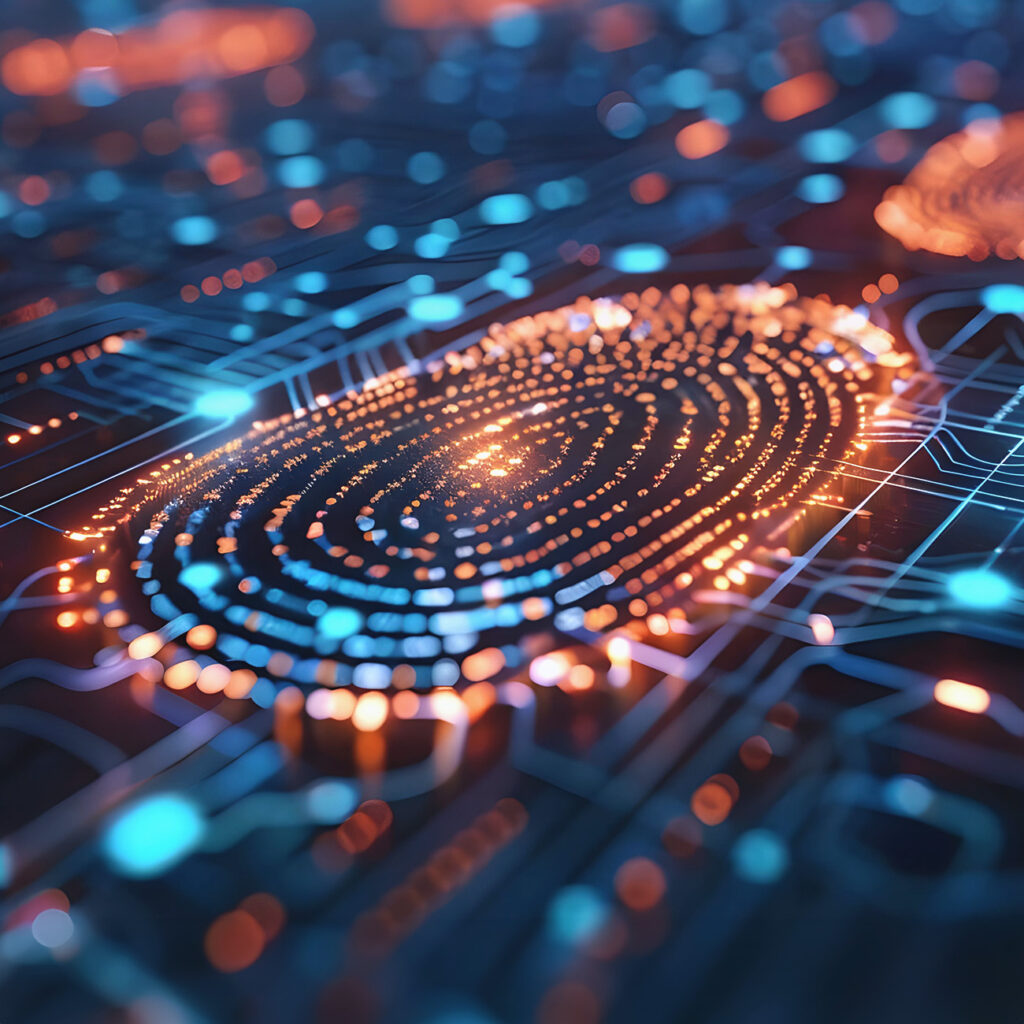
Data Transparency
Our ultimate goal is to establish a completely meta-data-driven certification process that executes testing procedures based on the characteristics of the learning method. The scientists of the Lamarr Institute acknowledge that data transparency is an important prerequisite for trustworthiness. Other key factors for the development of trustworthy solutions are privacy, bias, and fairness which are related to the interplay of data gathering, learning, and model exploitation.
Interpretable and Explainable Machine Learning
We aim at developing Machine Learning models that are interpretable and yield explainable results. Explanations need to be adjusted to the users’ specific needs, for instance by using methods of visualization or adding comprehensible documentation. In addition, AI systems must be verifiable. This can be facilitated by integrating expert knowledge or the results of simulations into the learning system. More traditional methods of program analysis and verification can be applied when AI systems are presented explicitly as code.
Testing for Robustness and Compliance
Finally, we are creating testing and certification procedures which include perturbations and adversarial attacks to evaluate robustness. We continue to collect and prepare benchmark data, assess resource consumption, and test compliance of an implemented pipeline with Machine Learning theory. Turning proofs into test procedures is a challenge for future research. An aspect of both verification and certification of growing importance pertains to ensuring compliance of AI systems with regulatory frameworks.