Embodied Artificial Intelligence
Embodied Artificial Intelligence refers to AI that is embedded in physical systems, such as robots, and can interact with the surroundings.
In contrast to classic Machine Learning in robotics, embodied AI encapsulates all aspects of interacting and learning in an environment: from perception, via understanding, reasoning, and planning to execution respectively manipulation. Just as human learning is based on exploration and interaction with the environment, embodied agents must improve their behavior from experience. Thus, embodied AI brings together multiple fields, such as computer vision, environment modeling, and prediction, planning, and control, reinforcement learning, physics-based simulation, and robotics.
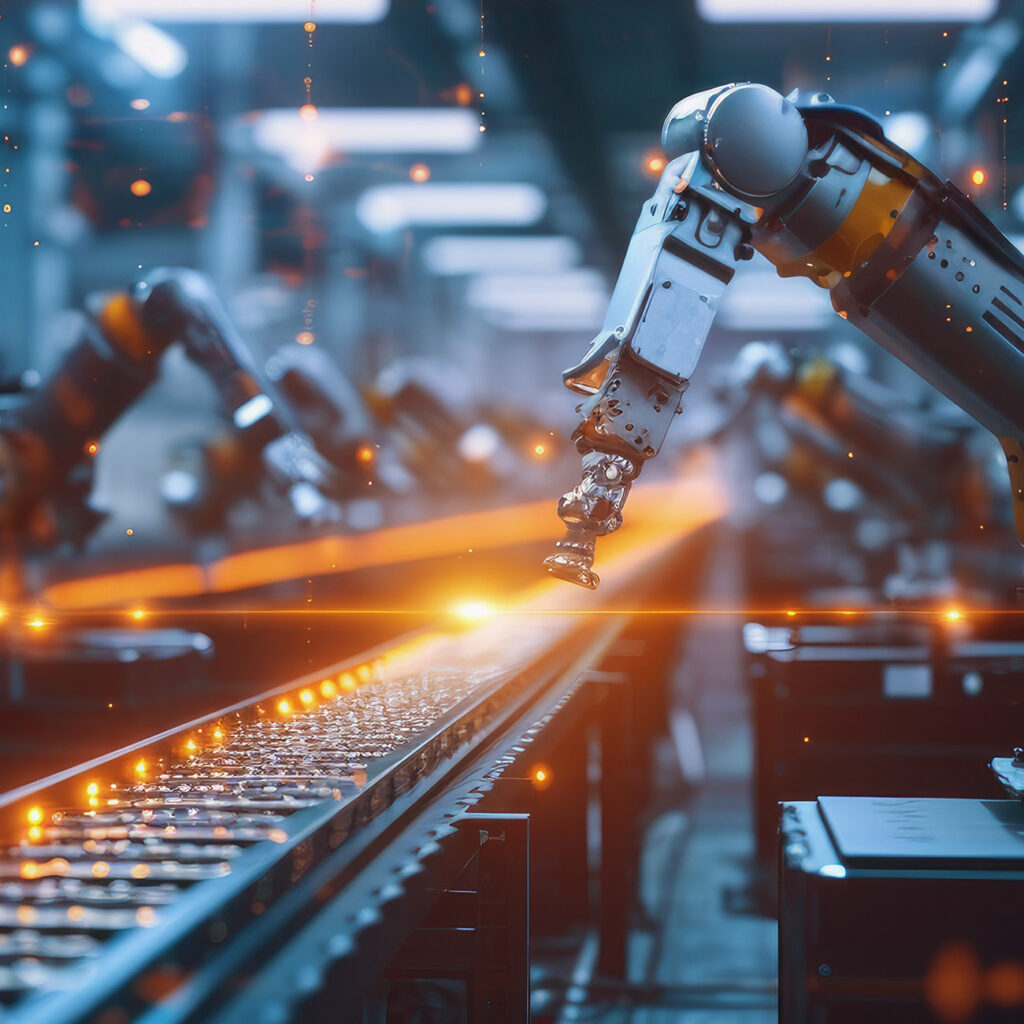
AI Applications for Machines
At present, AI research is highly data driven. The revolutionary breakthrough of Deep Learning consequently happened in application areas where data is readily available or can easily be generated. This makes sense in cyber areas, such as the internet, which by itself is a source of tremendous amounts of data. Looking at Europe and especially Germany, our societies’ success is strongly based on engineering and robotics and focusses heavily on manufacturing as well as logistics. As a result, there is a strong need to focus more on AI application for machines.
Embodied Intelligent Agents
Consequently, research on AI for machines needs a paradigm shift towards embodied AI. In the real world, intelligence should not exist in isolation; instead, it manifests itself though rich, multimodal interaction with the environment. So, an intelligent agent cannot be seen solely as a cyber component, but as an agent embodying the machinery. For such an embodied intelligent agent, its physical structure and properties, its sensor equipment, and its actuation capabilities play a critical role.
Learning and Interaction
In line with the AI3 paradigm, our approach to advance embodied AI is to add suitable structure to deep reinforcement learning. In this way, perception and planning of complex agent-environment interaction can be learned from limited experience. Simulation will be used to generate a digital reality enabling the agent to learn, relearn, and validate. Accordingly, embodied AI is not only of theoretical interest but of relevance to many real-world applications. Indeed, it concerns many of the application areas investigated at the Lamarr Institute, such as Natural Language Processing (NLP), manufacturing, or logistics.