Natural Language Processing
The research area Natural Language Processing (NLP) at the Lamarr Institute is a central hub associating research teams who are pushing the boundaries of natural language understanding and generation technologies.
The new Machine Learning paradigm – pretraining large models for a wide variety of tasks and on large amounts of data, then specializing the models for downstream applications – has affected all application areas, with language technologies being at the forefront.
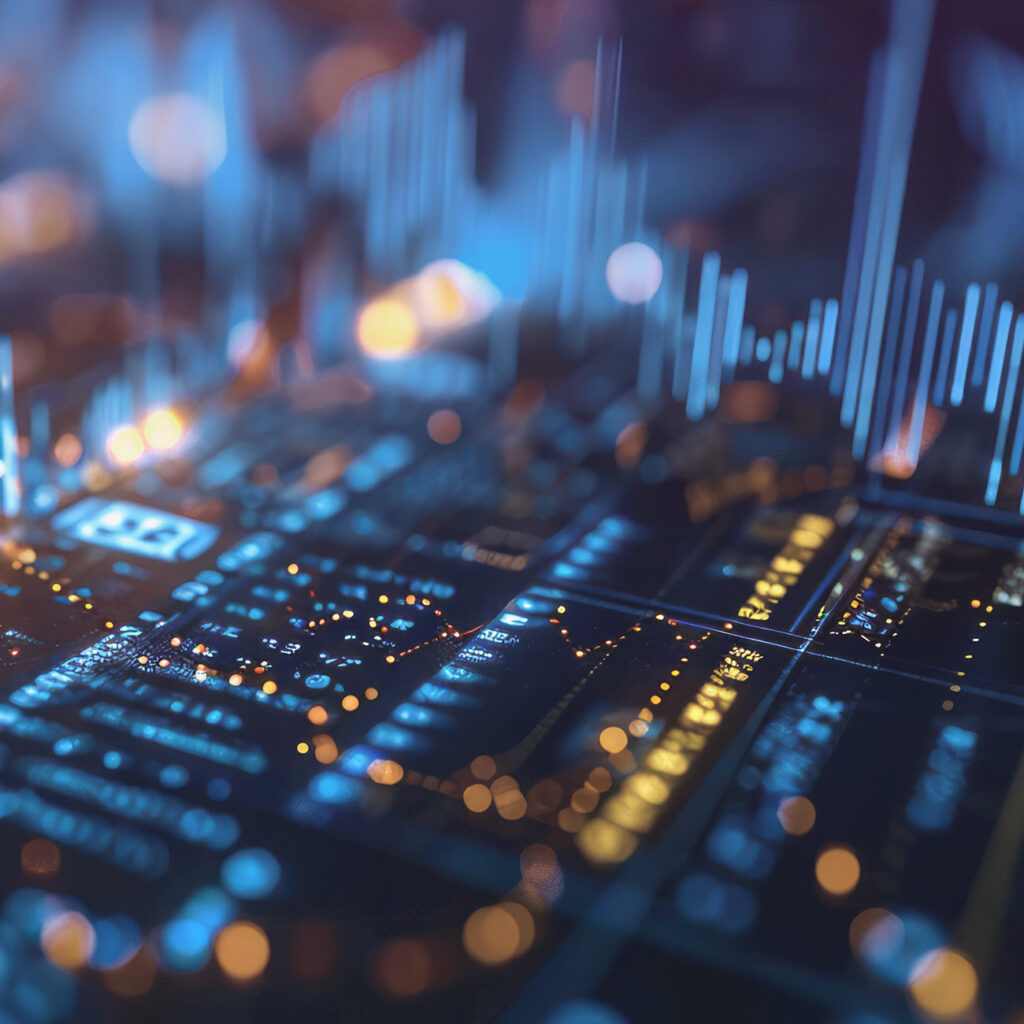
Critically Exploring Chances and Limitations of NLP Technologies
It is essential for the society to harness the power of large-scale language models, while understanding their risks and limitations and ensuring their safe, fair and ethical use. As researchers, we are committed to critically exploring the boundaries of new AI technologies in the language technology space, conducting thorough independent evaluations.
We are advancing methods that:
- make large language models (LLM) more robust to data problems so that we can learn more efficiently and reliably with less effort, as well as better serve underrepresented user groups,
- incorporate factual knowledge, advanced reasoning, and common sense, reducing generative hallucination issues
- enable more advanced personalization and perspective taking in order to produce more empathetic, safer and more supportive responses in a variety of social scenarios
- analyze and improve alignment of the LLMs with the human moral and ethical values
Contact persons
Fruitful Exchange with Research Community, Business and Society
Research on NLP at the Lamarr Institute is fundamentally shaped by the central paradigm of Triangular AI. While investigating the best algorithmic and architectural choices for such models, it is equally important to address data quality as well as representation of knowledge and context. At the same time, we focus on revisiting our existing evaluation approaches, and addressing the broader societal implications of such models. Together with our academic and industrial partners, we regularly organize events to stimulate discussions with top global researchers as well as the broad audience, supporting the society in the adoption of the new technology.