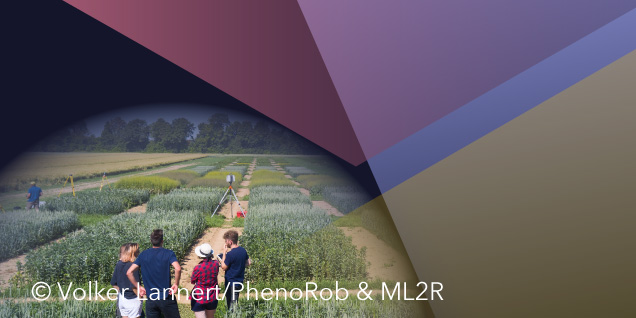
Crops play an essential role in the production of food and renewable raw materials. They are crucial for our society. However, crop production currently faces several challenges: the growing world population requires more food and, at the same time, more renewable resources for non-food products such as fuel. Therefore, we need to produce as much biomass from 2010 to 2050 as has been produced since the beginning of humanity—and do so sustainably. Yet, the available arable land and some of the nutrients needed by plants are limited. Additionally, we are already experiencing the negative effects of climate change, which will make the continuation of conventional agricultural production more difficult. Furthermore, unwanted impacts of agricultural production on the ecosystem, such as those caused using pesticides and fertilizers, need to be reduced to enable more sustainable management.
The Cluster of Excellence “PhenoRob—Robotics and Phenotyping for Sustainable Crop Production” at the University of Bonn uses a technology-driven and interdisciplinary approach to address these pressing real-world issues, and it is a global leader in research in the fields of robotics and phenotyping. Under the leadership of the scientific spokespersons Prof. Dr. Heiner Kuhlmann and Prof. Dr. Cyrill Stachniss, the cluster’s vision is to enable more productive, resource-efficient, and sustainable crop production by optimizing breeding and agricultural management through the development and deployment of new technologies. The PhenoRob team is interdisciplinary, comprising members from computer science, geodesy, robotics, plant science, soil science, agricultural economics, and environmental science. The cluster involves over 40 professors from both the Faculty of Agriculture and the Faculty of Mathematics and Natural Sciences at the University of Bonn. More than 70 young scientists are conducting research in six core projects on topics related to artificial intelligence (AI) and machine learning (ML), as well as their applications for more sustainable agriculture. PhenoRob is the only Cluster of Excellence in the field of agricultural sciences in Germany funded by the German Research Foundation (DFG).
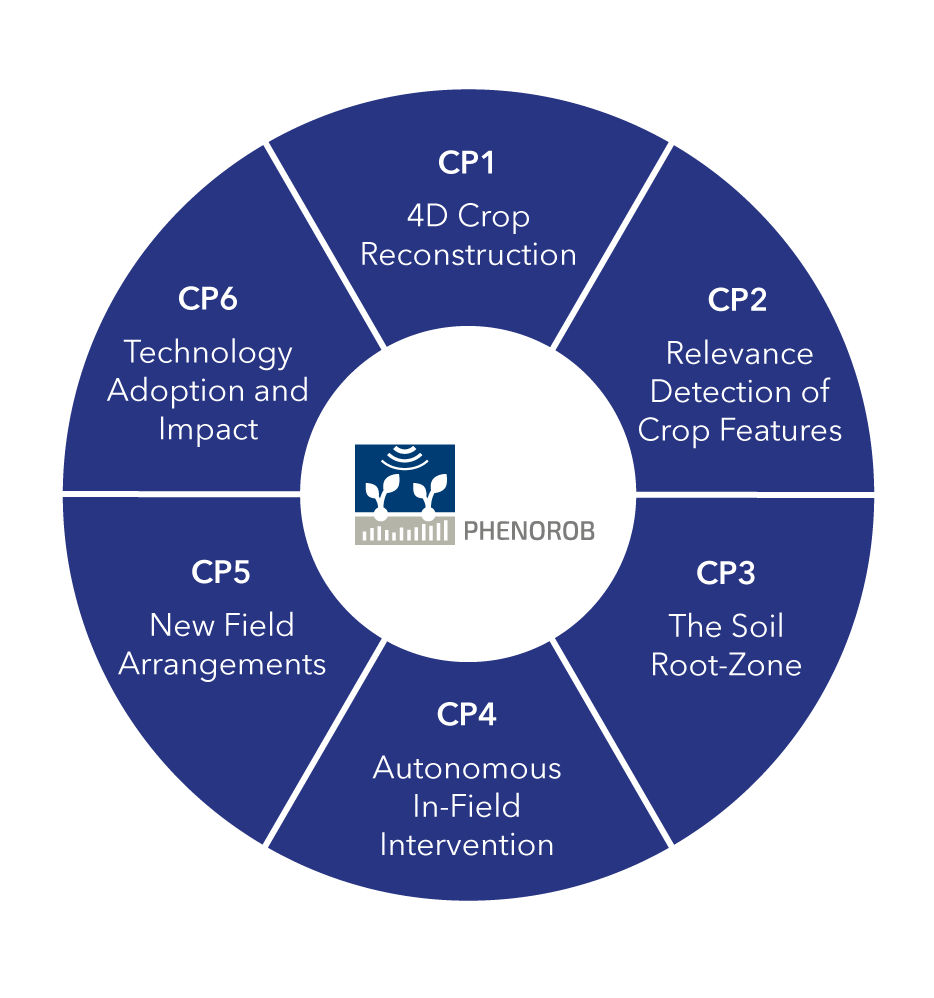
An overview of the six core projects of the cluster.
How Artificial Intelligence can revolutionize crop production
To increase crop yields while minimizing environmental impact in an economically viable manner, drastic changes in how we produce plants and significant advances in our scientific understanding are required. To achieve this, PhenoRob employs interdisciplinary methods from the fields of Artificial Intelligence and Machine Learning. Using various sensors, as well as ground robots and drones, the scientists monitor key aspects of crop production.
The various monitoring activities conducted in the projects generate large amounts of heterogeneous data about plants, plant populations, soil, and the environment. The researchers use these to analyze the extensive collected plant data. This helps improve AI and robotic understanding of plant growth processes, allowing them to better identify the relationships between inputs and outputs. For example, the connection between different growth stages and stress factors affecting yield development.
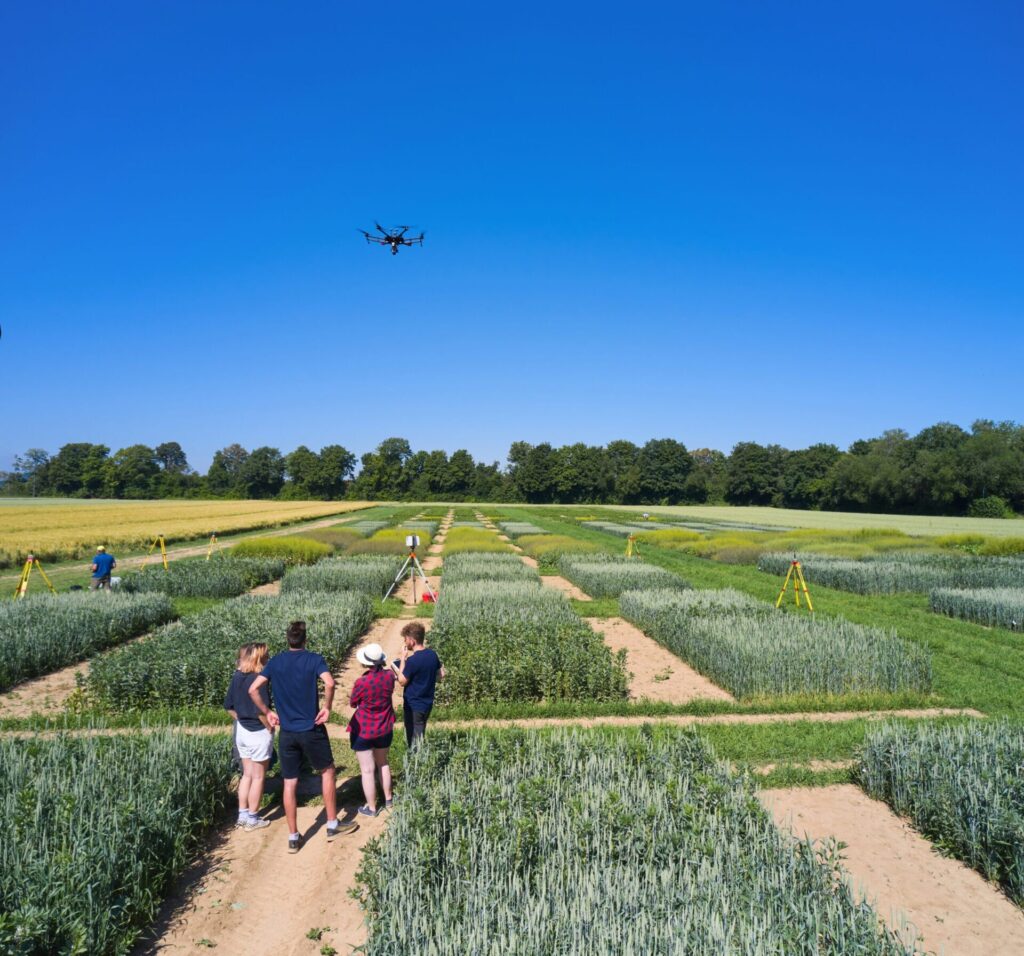
Researchers in the PhenoRob Cluster of Excellence use various technologies to monitor plants in the field.
Agriculture of the future: Two core projects as examples of AI and ML applications in the agricultural sector
Overall, there are six core projects within the Cluster of Excellence.
One of them is Core Project 4, “Autonomous In-Field Intervention.” Here, researchers are developing autonomous field robots that can identify individual weeds in real-time in the field. Precise, robot-assisted weed control reduces the use of chemicals like herbicides since weeds can be targeted instead of treated over large areas. Furthermore, it is possible to selectively determine which weed to combat, when, and how. Identifying the type of weed using modern learning algorithms, which combine scientific insights from plant research with new methods from computer science, allows the robot to apply the optimal treatment method: This could involve chemicals, a thermal or mechanical intervention, or even no intervention at all. These alternative, minimally invasive intervention techniques can prevent the development of herbicide resistance in key weed species.
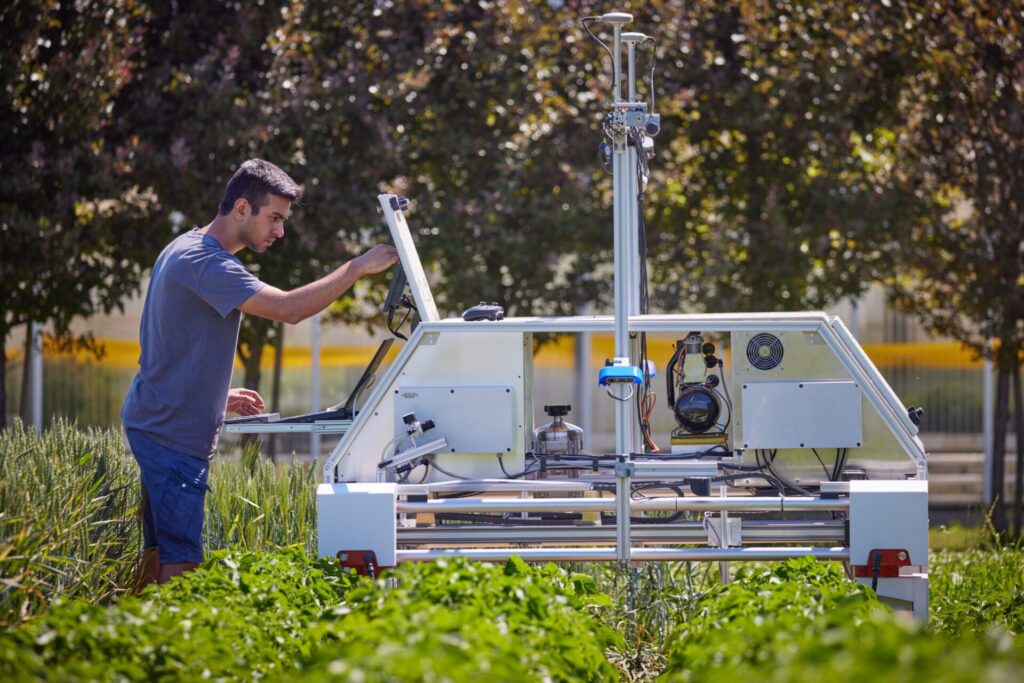
The PhenoRob robots are used in the field – one of their aims is autonomous weed control.
Another endeavor in Core Project 2, “Relevance Detection of Crop Features,” focuses on identifying previously unknown correlations between traits that affect plant development and yield. The interactions between plants and various types of stress, such as diseases, nutrient deficiencies, or drought, are central to this research. Connections between different growth stages and stress factors influencing yield development are investigated. Since nutrient deficiency symptoms on plants, for instance, are often not visible to the human eye until significant damage has occurred, scientists systematically analyze numerous early, relevant signs of nutrient deficiency and their detectability in multispectral sensor data. A series of experiments is conducted, and plant conditions are monitored to obtain a large amount of image data. These data are used to develop new algorithms capable of automatically detecting, predicting, and distinguishing specific nutrient deficiencies from other stressors.