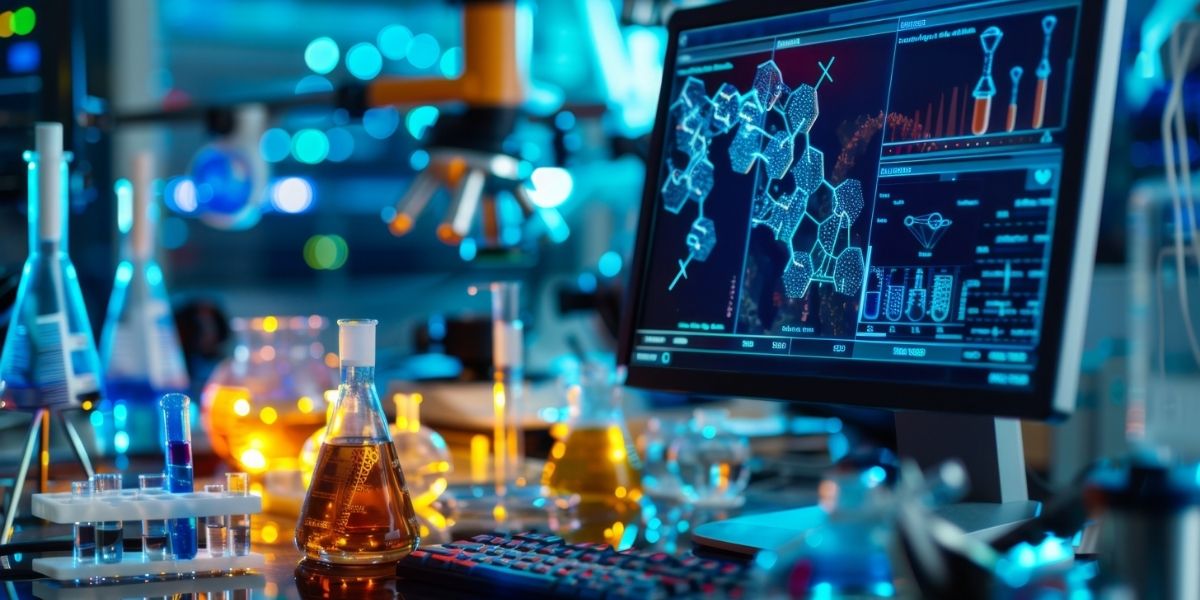
The scientific area “AI in Life Sciences” is a highly interdisciplinary component of the research at the Lamarr Institute, primarily focusing on Machine Learning (ML) in the life sciences including chemistry, and drug discovery, as well as leveraging concepts from Explainable Artificial Intelligence (XAI) to rationalize predictions. Research in this area aims to drive AI innovation and applications in interdisciplinary life science research and enhance scientific discovery and knowledge across different life science disciplines. In this blog post, we will introduce how Lamarr researchers are implementing AI and ML in Life Sciences.
Our approach aligns with the key guiding principle of the Lamarr Institute Triangular AI. By focusing on ML and XAI, the AI in Life Sciences area addresses the challenge of understanding the complex, ‘black box’ decision making processes behind ML models, which is crucial for advancing the life sciences for two key reasons:
- To build trust and transparency for non-experts – ML models and their predictions need to be trustworthy and understandable, especially to non-experts such as experimental investigators who rely on these predictions for experimental design. Without clear explanations of AI-driven predictions, skepticism, hesitance, and reluctance to trust computational findings are often prevalent. This is particularly true in fields like personalized medicine or drug discovery, where decisions can significantly impact people’s lives or involve substantial financial investments.
- To advance scientific knowledge – In interdisciplinary settings, AI should also contribute to the advancement of scientific knowledge and the understanding of complex, context-dependent tasks, such as exploring biological or chemical mechanisms. One of the key challenges in the research area is bridging the expertise of practitioners and AI specialists, fostering communication and interaction to drive scientific progress.
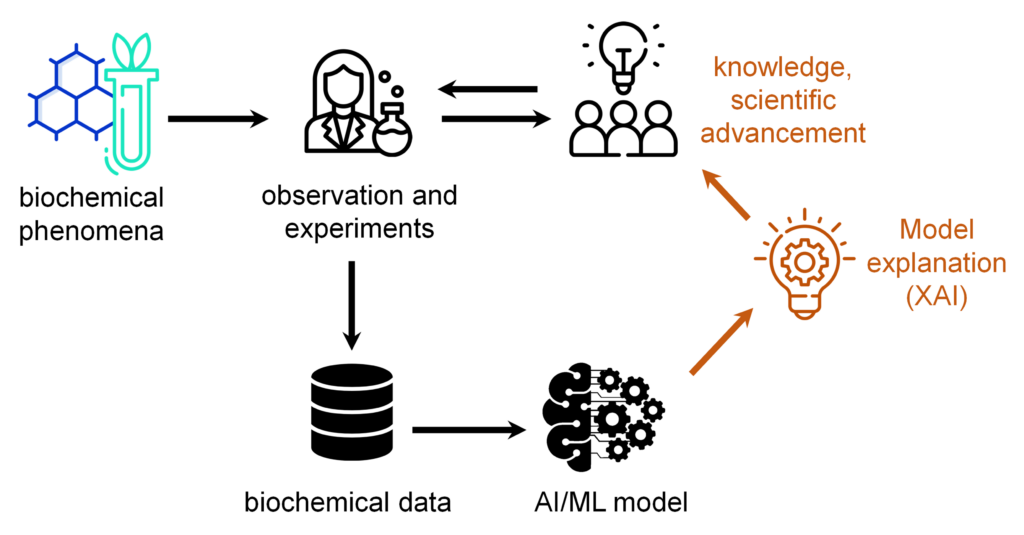
Computer-aided drug discovery
The Life Science Informatics (LSI) department at b-it, led by Prof. Jürgen Bajorath, is one of the key pillars of the Life Sciences area at the Lamarr Institute. The department’s primary focus lies in the development and application of ML and XAI methods and models for computational medicinal chemistry and computer-aided drug discovery. The central subjects of this research are small molecular ligands – substances like cofactors, metabolites, or drug candidates – that binds specifically to biological macromolecules, primarily pharmaceutically relevant proteins, to inhibit or enhance its activity or function.
Small molecules are computationally represented in various forms, such as molecular graphs, binary vectors (known as molecular fingerprints), textual representations (character strings), or images. These diverse representations serve as input for different (Deep Learning) models with varying computational architectures, enabling the learning of chemical structure-property relationships. This method can be applied for both generative and predictive molecular design applications.
Main research projects
One of the current major projects in the AI in Life Sciences area is the collaboration with the TüCAD2 Academic Drug Discovery Center at the University of Tübingen, focusing on protein kinase drug discovery and in particular in exploring the so-called “dark kinome”, which represents under-studied human kinases. Protein kinases are enzymes that catalyze phosphorylation of other proteins (that is, transfer of a phosphate group to side chains of tyrosine, serine, or threonine residues). The phosphorylation state of a protein often regulates its activity. Thus, kinases are critically important in signal transduction and regulation of cellular mechanisms. A key objective of the project with TüCAD2 is the identification of new active compounds targeting dark kinases to better understand their roles in intracellular signaling and their potential involvement in pathologies such as cancer or immunological diseases. This project exemplifies the close interactions between large-scale compound data analysis, predictive modeling, medicinal chemistry, and pharmacology.
Another major research focus of the research area is the development and application of“biochemical language models” based on transformer architectures. These specialized language models leverage textual representations of small molecules and/or proteins, enabling predictive tasks that were previously challenging or impossible to address using traditional ML methods: for instance, designing new active compounds based on biological sequence data or identifying compounds with increased potency or different selectivity has been a longstanding challenge for researchers. Furthermore, the generation of multi-target compounds (i.e. compounds that can selectively bind to more than one target protein) is a highly relevant application of these type of transformers. The ability to bind to multiple target proteins is often desirable in drug discovery since the treatment of various pathologies including cancer benefits from simultaneous interference with two or more proteins. Recently, we have developed a transformer model that takes as input single-target compounds (i.e. compounds that bind only one protein) and generates as output dual-target compounds with desired activity (i.e. compounds that bind two different proteins).
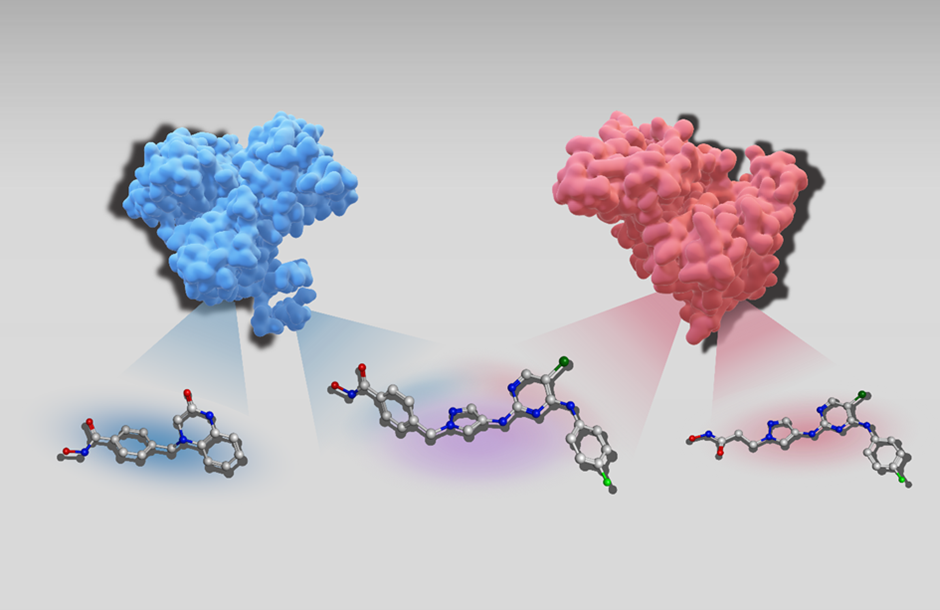
Schematic representation of AI-generated dual target compound vs single target ones
XAI approaches also play a crucial role in the AI in Life Sciences area at Lamarr, as highlighted earlier. Here, new concepts and methods are being developed to not only understand how ML models arrive at specific predictions (level 1) but also to interpret these predictions from a chemical or biological perspective (level 2). For example, in a recent project learning characteristics of graph neural networks (GNNs) used in drug design have been thoroughly investigated using newly developed methodology called EdgeSHAPer. This method leverages Shapley Values from cooperative game theory to assess the importance of specific edges in GNN predictions, providing deeper insight into feature relevance.
The dual-level explanation of predictions also enables researchers to investigate causality relationships between ML model predictions and the chemical or biological processes they learn, such as the targeted inhibition of protein with newly designed compounds. This exploration of causality naturally intersects with human reasoning, opening avenues to connect AI research with cognitive sciences and philosophical concepts. This interdisciplinary perspective represents an opportunity for growth in the AI in Life Sciences areas, extending beyond the core disciplines of life sciences.
Find out more about Lamarr’s research area of AI in Life Sciences here.