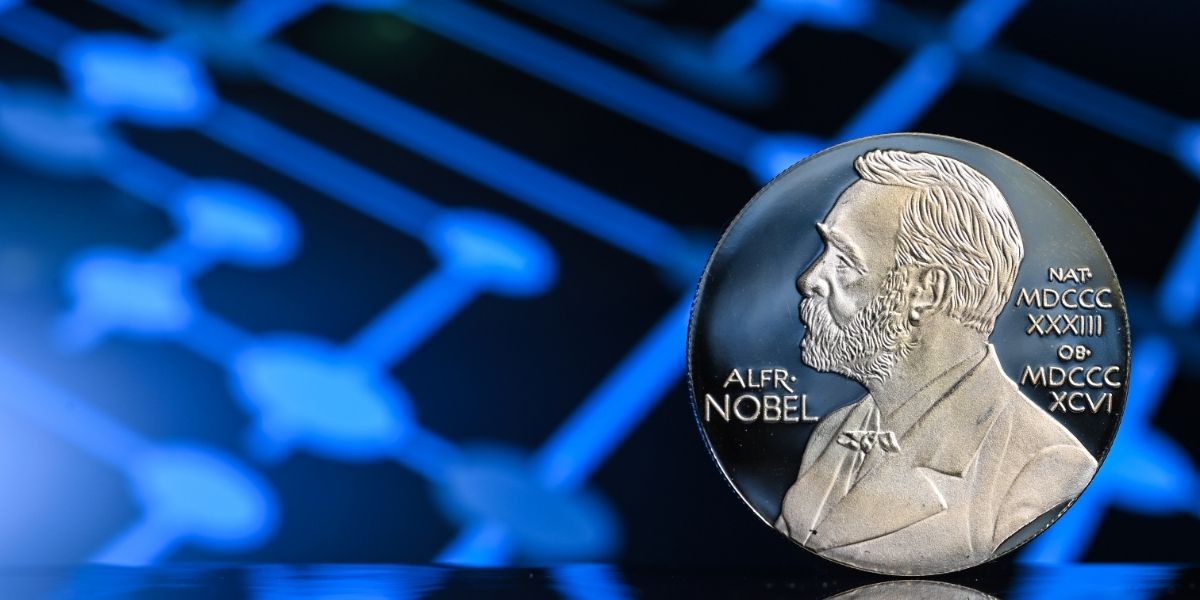
In this blog post, I share some of my thoughts about this year’s Nobel Prize in Physics which surprised me as much as everybody else. I reflect on what the laureates are being honored for and argue that it is more than justifiable to award a Nobel Prize for research in artificial intelligence whose practical applications are currently revolutionizing many aspects of our daily lives.
If you are reading this blog, you likely already know that the 2024 Nobel Prize in Physics has been awarded to John Hopfield and Geoffrey Hinton. To see why, let me just quote from the press release of the Nobel Committee for Physics: “When we talk about artificial intelligence, we often mean machine learning using artificial neural networks. This technology was originally inspired by the structure of the brain. In an artificial neural network, the brain’s neurons are represented by nodes that have different values. These nodes influence each other through connections that can be likened to synapses […]. This year’s laureates have conducted important work with artificial neural networks from the 1980s onward.”
Looking at the Committee’s decision, it is fair to say that nobody had expected it. (I should know because, in the week prior to the announcement, I watched several videos by physics YouTubers with predictions for this year’s prize and nobody had artificial intelligence (AI) researchers on their lists.)
It is also fair to say that the decision is somewhat controversial. On the one hand, there are physicists who criticize that the prize goes to computer scientists and see this as an indicator of a crisis in physics. On the other hand, there are computer scientists who see the prize as an attempt by physicists to claim AI as their discipline. (Just google it 😉.) There also is some controversy regarding the prize-worthiness of the laureates and whether the contributions they are awarded for are wrongly attributed. (Just google it 😉.)
In the following, I will address all this and discuss why Hopfield and Hinton indeed did fundamental work on AI which bridges computer science and physics. In doing so, I shall assume that readers know what artificial neural networks are, namely mathematical models of biological neural networks that can be implemented on computers to perform a wide variety of cognitive tasks. So, here we go …
Invention of Artificial Neural Networks
Contrary to what we now read in the news, Hopfield and Hinton did not invent artificial neural networks and never claimed to have done so. In fact, artificial neural networks date back at least to the 1940s when the first electronic computers became available, and people started thinking about how to simulate human thought processes with “electronic brains”.
Indeed, the first concept of an electronic neuron was described by McCulloch and Pitts in 1943 who also discussed how to do logic computations with networks of such neurons [1]. In the 1950s, Rosenblatt built an electronic neuron which he called a perceptron and demonstrated that it can learn to recognize patterns using training data and an algorithm due to Hebb [2,3]. Networks in which perceptrons are stacked in layers and only pass information from one layer to the next came to be known as feed-forward multilayer perceptrons and were the precursor of many of today’s deep neural networks. Neural networks in which perceptrons are not necessarily arranged in layers and in which information can flow back and forth came to be known as recurrent neural networks and they, too, play a fundamental role in modern deep learning.
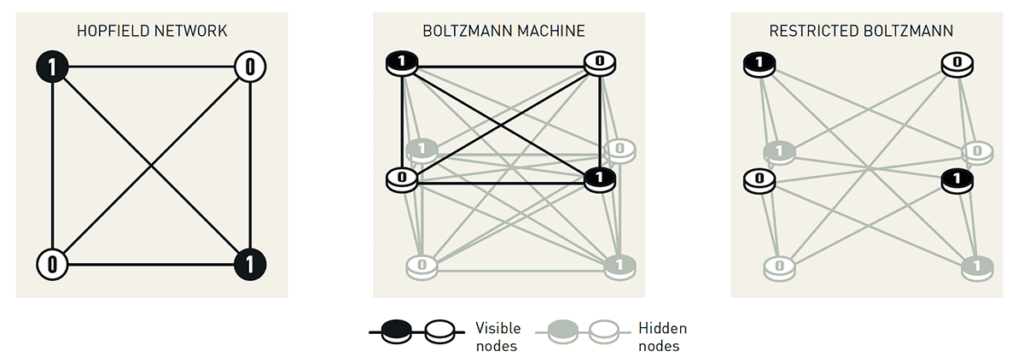
Bridging Computer Science and Physics: Contributions of Hopfield and Hinton to AI Research
Given this historical context, I can now discuss the contributions of Hopfield and Hinton and their central role for much of the ongoing efforts in artificial intelligence research.
In the 1970s, Little and Amari independently conceptualized a kind of recurrent neural networks which are now known as Hopfield nets [4,5]. At first sight, this attribution seems surprising because John Hopfield acknowledged that he considered other people’s ideas when he extended them a few years later [6]. However, as a physicist (not a computer scientist as we now often read), Hopfield had the fundamental insight that the activity of the neurons in Hopfield nets can be understood as an energy minimization process. In other words, he connected mathematical biology, mathematical psychology, and AI to physics which has far reaching consequences.
Hopfield nets were originally conceived as a mathematical model of how the brain stores and retrieves patterns. That is, they were originally meant to describe memories. After Hopfield published his work, the connection between memory formation and physical phenomena caused quite some excitement which later receded when it was found that original Hopfield nets are not very good at memorization [7]. However, Hopfield himself pointed out yet another, curiously underappreciated application of Hopfield nets, namely their use as constraint satisfaction- and combinatorial problem-solving machines [8].
I will not go into the details of this latter aspect of Hopfield nets because they are quite technical. But I will point out that this aspect of Hopfield nets is of considerable current interest as it allows for building bridges between AI and quantum computing due to the insights we owe to Hopfield. At the Lamarr Institute, we are actively working in this exiting area ourselves [9,10]. To showcase our ongoing research on quantum AI, I am delighted to point to recent work by Sascha Mücke who uses Hopfield inspired models to solve Sudokus on quantum annealers [11]. Note that “inspired” is the exact right term because (by now unsurprisingly) Hopfield himself already showed how neural networks can solve these kind of puzzles [12].
Geoffrey Hinton is often called “the godfather of AI” and I assume that most readers of this blog will have heard of him and his work. However, as he is a computer scientist one might wonder why he would receive the Nobel Prize in Physics.
While he is arguably most famous as one of the discoverers and popularizers of the error backpropagation algorithm [13] which allows for training multilayer perceptrons (and has roots and precursors reaching further back [14]), the Nobel Committee cites his earlier contributions to theory and applications of Boltzmann machines [15,16]. These extend and generalize Hopfield nets using concepts from statistical physics and played a crucial role in the development of what we now call deep learning. Indeed, in the late 2000s, Hinton and his colleagues worked with what they called deep Boltzmann machines to perform simple image classification tasks [17]. Their ideas then quickly led to deep convolutional neural networks for much more advanced image classification tasks and to the publication which arguably kick-started the deep learning revolution as it shattered any remaining concerns as to the capabilities of artificial neural networks [18].
Current and Future Impact of the Nobel Laureates’ Research
When elaborating on the scientific background to this year’s Nobel Prize, the Nobel Committee cited a wide range of application areas for artificial neural networks in physics and other scientific disciplines. In this context, the IceCube neutrino detector project at the South Pole was mentioned [19]. The project resulted in the first neutrino image of the Milky Way, a breakthrough which was achieved by TU Dortmund University educated scientist Mirco Hünnefeld in collaboration with the research groups of Lamarr Institute founding director Katharina Morik and Lamarr Area Chair Physics Wolfgang Rhode.
All in all, I therefore think that the Nobel Prize in Physics for Hopfield and Hinton is well deserved. Both applied methods from the physics toolbox to understand and improve the behavior of the kind of models which are now driving the AI revolution. Moreover, their work from the 1980s is still shaping today’s research landscape and therefore is likely to enable even further breakthroughs.
References
[1] W.S. McCulloch and W. Pitts. A Logical Calculus of the Ideas Immanent in Nervous Activity. Bulletin of Mathematical Biophysics, 5(4). 1943.
[2] F. Rosenblatt. The Perceptron: A Probabilistic Model for Information Storage and Organization in the Brain. Psychological Review, 65(6). 1943.
[3] D.O. Hebb. The Organization of Behavior. Wiley. 1949.
[4] W.A. Little. The Existence of Persistent States in the Brain. Mathematical Bioscience, 19(1–2). 1974.
[5] S.-I. Amari. Neural Theory of Association and Concept-formation. Biological Cybernetics, 26(3). 1977.
[6] J.J. Hopfield. Neural Networks and Physical Systems with Collective Computational Abilities. PNAS, 79(8). 1982.
[7] D.J. Amit, H. Gutfreund, and H. Sompolinsky. Information Storage in Neural Networks with Low Levels of Activity. Physical Review A, 35. 1987.
[8] J.J. Hopfield and D.W. Tank. “Neural” Computation of Decisions in Optimization Problems. Biological Cybernetics, 52. 1985.
[9] C. Bauckhage, R. Sanchez, and R. Sifa. Problem Solving with Hopfield Networks and Adiabatic Quantum Computing. In Proceedings of International Joint Conference on Neural Networks. 2020.
[10] C. Bauckhage, R. Ramamurthy, and R. Sifa. Hopfield Networks for Vector Quantization. In Proceedings of International Conference on Artificial Neural Networks. 2020.
[11] S. Mücke. A Simple QUBO Formulation of Sudoku. In Proceedings of the Genetic and Evolutionary Computing Conference Companion. 2024.
[12] J.J. Hopfield. Searching for Memories, Sudoku, Implicit Check Bits, and the Iterative Use of Not-Always-Correct Rapid Neural Computation. Neural Computation, 20(5). 2008.
[13] D.E. Rummelhart, G.E. Hinton, and R.J. Williams. Learning Representations by Back-propagating Errors. Nature, 323(6088). 1986.
[14] J. Schmidhuber. Deep Learning in Neural Networks: An Overview. Neural Networks, 61. 2015.
[15] G.E. Hinton, T.J. Sejnowski, and D.H. Ackley. Boltzmann Machines: Constraint Satisfaction Networks that Learn. Technical Report CMU-CS-84-119. 1984.
[16] D.H. Ackley, G.E. Hinton, and T.J. Sejnowski. A Learning Algorithm for Boltzmann Machines. Cognitive Science, 9(1). 1985.
[17] R. Salakhutdinov and G.E. Hinton. Deep Boltzmann Machines. In Proceedings of International Conference on Artificial Intelligence and Statistics. 2009.
[18] A. Krizhevsky, I. Sutskever, and G. Hinton. 2012. ImageNet Classification with Deep Convolutional Neural Networks. In Proceedings of Advances in Neural Information Processing Systems. 2012.