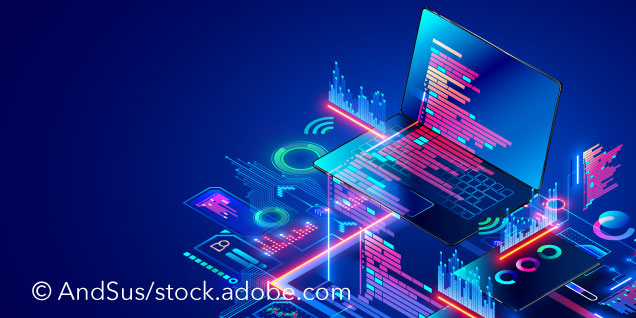
Especially in recent years, interest in the field of Machine Learning has significantly increased, and substantial progress has been made. The overarching goal: approaching Artificial General Intelligence (AGI), which is a system possessing competencies comparable to the cognitive abilities of a human, allowing it to understand and solve any kind of problem. However, with the advancing development, concerns about the misuse or misappropriation of AI systems are growing, and, with that, there is an increasing need for appropriate ethical guidelines. Some time ago, the focus was particularly on the language model GPT-2 by the research institution OpenAI, which caused a sensation with its high-quality and exceptionally human-like text generation capabilities. The fear underlying this: the use of the model to mimic important public figures and the automated generation of fake news. But are these concerns justified? And how far do the capabilities of AI models actually go?
How was GPT-2 trained?
GPT-2 belongs to the series of Generative Pre-trained Transformers (GPT), developed by researchers at OpenAI. As the name suggests, GPTs focus not only on basic language understanding but also on text generation. In its architecture, GPT-2 is similar to other Transformer models like BERT or ELMo but uses only decoder blocks instead of encoders and operates auto-regressively (for more information on how BERT works, check the blog post “BERT: How Do Vectors Accurately Describe the Meaning of Words?”). Another peculiarity lies in the size of the model. The individual model variants comprise up to trillions of parameters, many times more than other Transformer architectures, such as BERT Large with 340 million parameters.
In training, an unsupervised approach with a generalized optimization function was chosen for GPT-2. This means there was no explicit training problem; the model simply had to learn the distribution of words within a given context and thus gain a general understanding of language. A dataset consisting of text corpora from various domains or genres was used to bring more diversity into the model. The goal was to mimic human learning behavior, and GPT-2 was developed to be a “multi-task learner” capable of learning general patterns in linguistic input and later transferring them to various tasks. In contrast, many NLP problems currently follow an approach with task-specific learning or fine-tuning. While the GPT precursor still benefits from this approach, the trend is now shifting from so-called “narrow experts” towards “competent generalists” and more adaptable models.
The problem – AI in the wrong hands
With this novel perspective on Machine Learning, GPT-2 could compete with specialized models and even achieve state-of-the-art results on some benchmark datasets. It is important to keep in mind that GPT-2 had not seen these test datasets before and had to solve the required tasks purely based on natural language instructions. Astonishing results also emerged in text generation tasks: Given input examples, GPT-2 generates arbitrarily long continuations congruent with the thematic content. The model behaves “chameleon-like”, adapting to the given input in style and tone. This demonstrates an unprecedented level of language understanding and expressiveness.
However, it was precisely these capabilities that led to concerns among the public and developers. Perhaps the most well-known piece generated by GPT-2 is dedicated to the discovery of a herd of unicorns in the Andes. The generated text resembles a newspaper article in form and style, showcasing an unprecedented quality of output, especially in aspects of creativity, coherence, and argumentation. At first glance, it is not recognizable as automated and could be mistaken for the work of a human. Other examples include a report on stolen nuclear material and a testament to the alleged dangers of recycling. The concern is justified in the sense that third parties could use the language model to generate exactly these types of contributions, spread them through social media, and influence the masses. To counteract this, OpenAI decided to initially release only smaller model variants with lower performance.
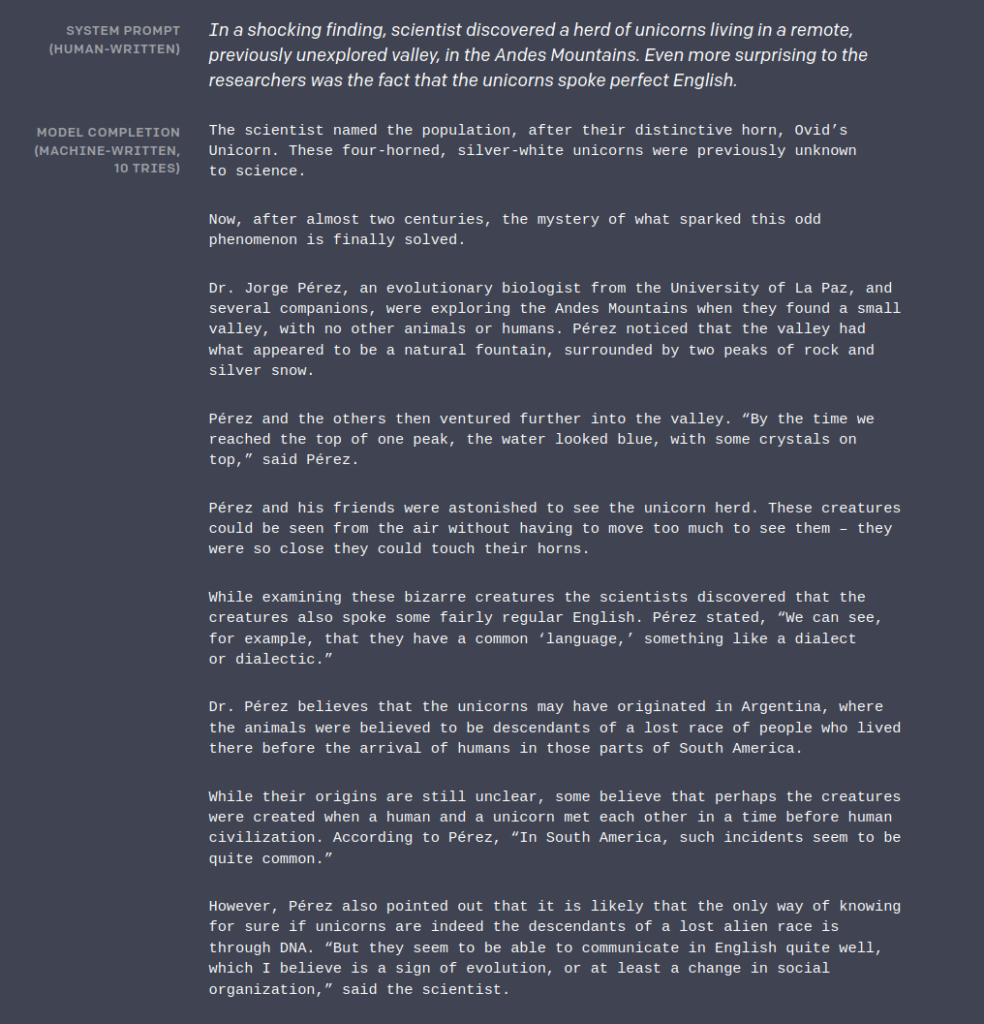
Excerpt from the text generated by GPT-2 on the discovery of talking unicorns in the Andes.
The reality – Studies and additional models
But how good is the model really? How close is it to human capabilities? According to assessments by the OpenAI team, the number of truly coherent and naturally sounding texts is limited to only about 50% of the outputs generated during initial tests. The aforementioned contributions belong to a selection of carefully curated examples, and their representativeness for the entirety of generated texts is questionable. The authors themselves note that it takes a few attempts to produce such a convincing post. Often, there are inconsistencies such as repetitions, sudden changes of topic, or issues with modeling the real world. Upon closer inspection, even the showcase examples exhibit minor incongruities: in the case of the unicorns, they are initially referred to as a species that evolved, but later referenced as an alien race. There are also discrepancies concerning spatial relationships.
Another factor influencing the quality of outputs is the model’s “familiarity” with the topic of the post to be generated, or in other words, the distribution of training data. Similar to other networks, it is easier for models to generate outputs that are very similar to the training data and frequently occur in the dataset. For text generation models, these are often forum posts, Wikipedia, and news articles, as these sources are readily available for new web crawl datasets. Other topics such as technology and esoterica, on the other hand, occur less frequently and are therefore more challenging to reproduce. Regarding creativity and text understanding, it becomes clear that GPT-2 is primarily still a simple language model, whose main task is only to calculate the probabilities of consecutive words.
As a final point, the size of the models seems crucial for their adaptability, and the number of parameters and performance appear to strongly correlate. Especially, the texts generated by the largest models are very difficult to differentiate from those of a real person. Contrary to the initial concerns, OpenAI has now also released these larger model variants and even made the models available for commercial purposes. A successor, GPT-3, is already available, focusing on generating Twitter-like texts. However, for studies on this model, the developers took a bit more time and conducted studies with human participants. In assessments of the authorship of a given text – human or machine – the participants’ recognition rate was approximately 52%. Unlike GPT-2, the Twitter posts generated by GPT-3 are much shorter and freer in structure, making them much easier to generate. In this context, the texts generated by GPT-2 are potentially easier to identify and likely less dangerous than initially assumed. The ultimate question is whether the models were initially withheld for publicity reasons or indeed due to concerns about their use.
More time for research
In summary, for the field of Natural Language Processing, the trend is moving towards simpler model architectures that gain versatility and quality in their representations with depth. There is also a strong dependence on the training data used, which means that even smaller models trained on specific datasets could produce concerning outputs. For future research, there should be increased emphasis on quality control of the available training data, and biases, controversial, and other questionable content should be filtered more rigorously. What OpenAI is right about in this regard is the call for more time for the development of AI models, including the aforementioned aspects, testing through qualitative and quantitative evaluation studies, and adherence to appropriate guidelines. This could help to better avoid inappropriate outputs and ensure the meaningful use of models like GPT-2. For more insights into Slow Science, check out the blog post “Slow Science: More time for research in Machine Learning”.