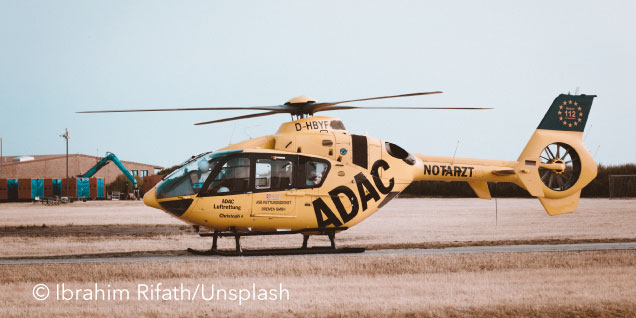
Artificial Intelligence is already an integral part of the medical context. Especially in times of a pandemic, digitization and streamlining of processes in healthcare can assist in saving valuable resources and mitigating overload, for example through the use of telemedicine applications such as digital consultations and intelligent surgical planning. This creates completely new potential – but also challenges – for the use of Artificial Intelligence.
Decision support in emergency care through Artificial Intelligence
In the Fraunhofer IAIS research project LOTTE (“Guidance System for Optimizing the Therapy of Traumatized Patients in Initial Treatment”, or in German, “Leitsystem zur Optimierung der Therapie traumatisierter Patienten bei der Erstbehandlung”), we have focused on the area of emergency care for severely injured patients. In Germany, there are more than 2.6 million traffic accidents annually, resulting in almost 400,000 injuries, including 68,000 severe injuries and more than 3,200 fatalities. In addition to severe injuries, there are work, school, sports, and recreational accidents, so statistically, 12% of the German population experiences an accident in a year. Severely injured individuals are transported to a hospital by an ambulance or rescue helicopter. There, critically injured individuals and so-called “polytrauma” patients, who have been multiply and life-threateningly injured, are immediately treated in a specially equipped trauma room.
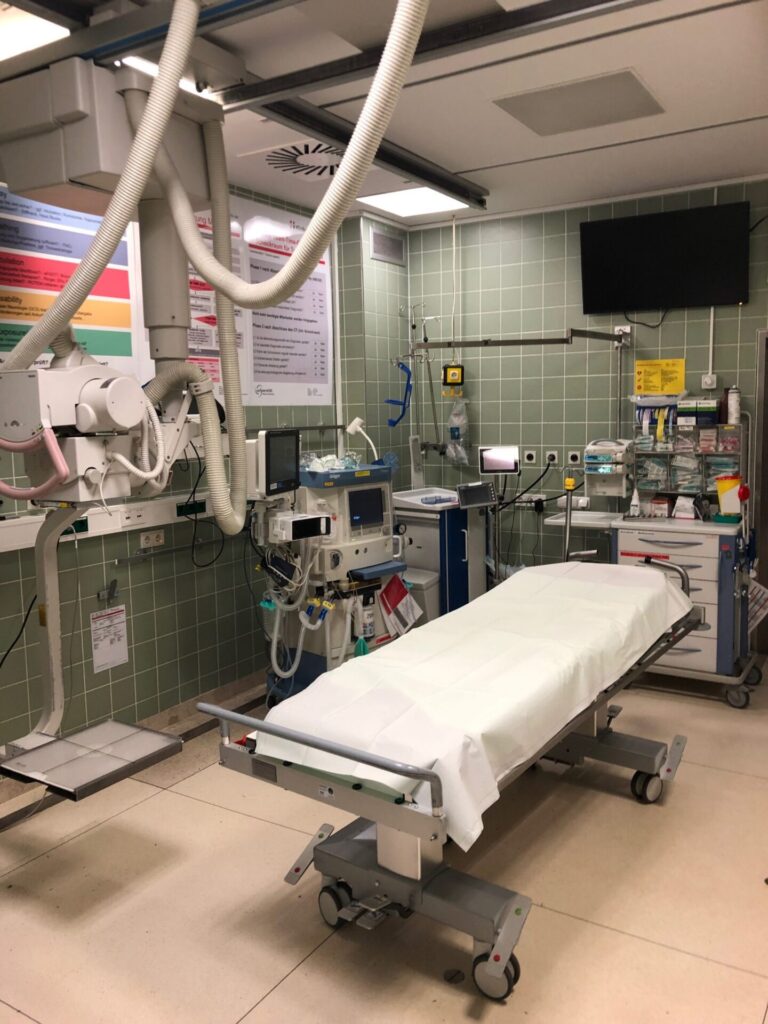
The trauma room is the central hub for the care of severely injured individuals in the hospital (Photo taken in the Merheim Hospital).
The goal of the project was to develop and evaluate scenarios for the use of Artificial Intelligence and big data to improve the quality of treatment. Together with our interdisciplinary partners in trauma surgery, health economics, and legal studies, we closely examined the data flows and decisions that play a role in the treatment of severely injured individuals. It quickly became clear that this area has several peculiarities that must be carefully considered in the development of AI systems.
Challenges in the treatment of severely injured individuals
The care of severely injured individuals is very complex and occurs under high time pressure. An entire team from trauma surgery, anesthesia, neurology, radiology, and nursing collectively decides on measures and interventions. Nevertheless, approximately 14% of all severely injured individuals in Germany succumb to their injuries.
The amount of continuously new information of various kinds, the necessity of medical interventions, and the high temporal dynamics and uncertainty make decisions for the trauma room team challenging. In detail, the following focal points can be identified:
- Data situation: During the treatment of a severely injured person, the trauma room team must process a large amount of diverse data on the accident and vital status to make decisions.
- Communication: A diverse team from trauma surgery, anesthesia, neurology, radiology, and nursing decides collectively on measures and interventions.
- Time intensity: Decisions must be made under time pressure to prevent permanent damage or the death of the injured individuals.
Approaches for Artificial Intelligence methods
In the context of the LOTTE project, six scenarios were developed, which target different points along the treatment path, and which integrate various Machine Learning technology architectures:
- Intelligent alarm chain: The phone call between the paramedic and the control center is automatically converted into records and made available to the trauma room team before the patients arrive. Procedures from “Natural Language Processing” (NLP) are used for this purpose.
- Semi-automatic speech documentation: To avoid any loss of information during the handover from paramedic to the trauma room team, a system records the handover and automatically creates a structured protocol.
- Trajectory classification: The system calculates an objective and quantitative assessment of the complexity of the case at hand and the expected course. The result is retrieved by the trauma room leadership and digitally visualized for the entire trauma room team.
- Smart guideline interface: After entering case parameters, the system provides a visualization of the guideline-compliant treatment process as well as case-specific treatment recommendations.
- Literature mining: This is a semantic search for the countless documents in medical literature. By entering case parameters, the team can research information about rare or unknown disease constellations.
- Surgical risk assessment: Before a possible surgery, the individual risk of patients for several types of complications (such as sepsis or acute kidney failure) is calculated, thereby supporting decision-making for the undertaking of operative measures.
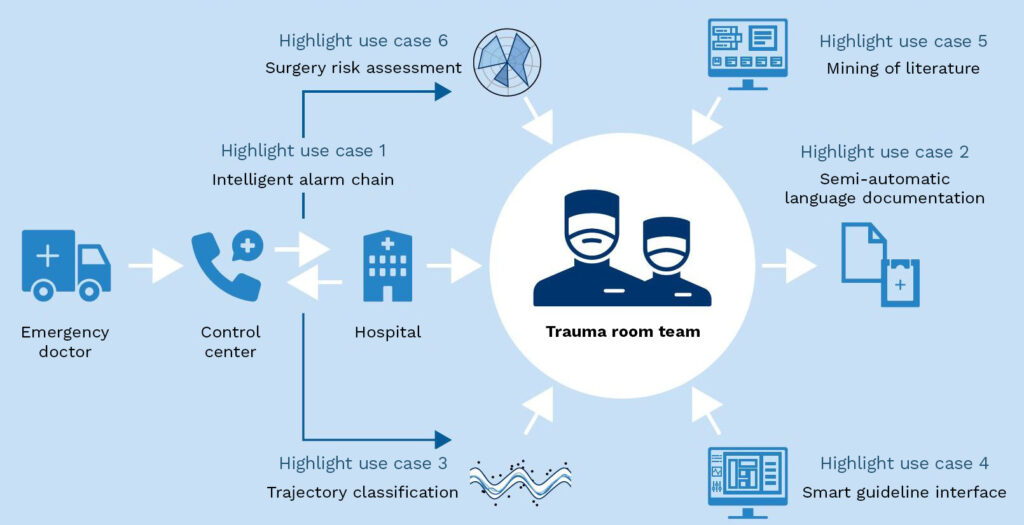
Overview of the presented usage scenarios along the treatment path in the care of severely injured individuals
“KI Absicherung” (AI Security) and legal issues
The implementation of Artificial Intelligence systems in the medical context brings not only significant opportunities but also new challenges. For every software used in a medical environment, data protection and functional safeguarding must be considered. Additionally, the suggestions of an automatic system for users should be comprehensible so that errors or disadvantages can be detected and avoided early on. If the use of AI systems becomes commonplace, an “automation bias” must also be prevented, where too much trust in the system prevents a critical review of the outputs (see also the blog post: The path to secure AI).
Future significance of Artificial Intelligence in hospitals
The extraordinary societal and political changes in healthcare, including demographic changes, increased staffing shortages, and higher patient demands, can only be overcome with the help of digital solutions. Personalized medicine, rare diseases, and population growth further change the daily work of qualified staff. Especially hospitals have a special task in the emergency care of severely injured individuals and must address this in the future through intelligent technological support. In the long term, the healthcare system must strategically and structurally position itself so that AI methods are meaningfully and confidently integrated into workflows, considering both patients and staff.
Project Partners: We would like to thank our project partners for their collaboration: The Chair of Management and Innovation in Healthcare and the Chair of Trauma Surgery and Orthopedics at the University of Witten/Herdecke, as well as the Institute of Legal Informatics (IRI) at Leibniz University Hannover. We also thank Merheim Hospital of the Cologne Clinics for their support.
More information in the associated publication:
D. Antweiler, K. Beckh, J. Sander, S. Rüping: Künstliche Intelligenz im Krankenhaus: Potenziale und Herausforderungen – Eine Fallstudie im Bereich der Notfallversorgung, 2020, PDF.