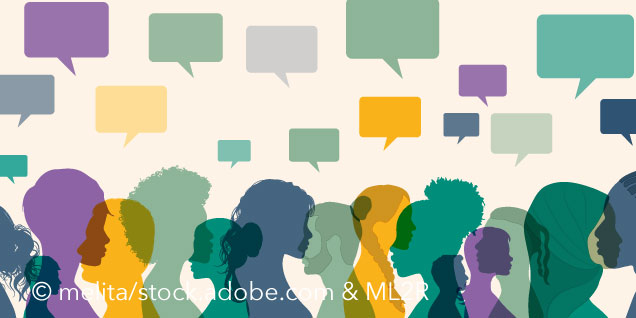
In today’s world, audiovisual data is indispensable for many professional groups. For journalists, editors, researchers of various disciplines, content creators, museum staff, and archivists, this data forms a crucial part of their daily work. The rapid growth of public, but also internal, access-restricted audiovisual databases has presented these groups of people with ever greater challenges in recent years.
The use of such data through Artificial Intelligence (AI) methods has been simplifying the work of some of these professions for several years—for instance, by enabling text search in videos using automatic speech recognition. However, the potential for audiovisual search is far from exhausted.
Search in audiovisual data collections
AI-driven approaches for audiovisual analyses have, due to their immense capabilities, enabled the exploration and searchability of extensive data collections in recent years. An example of this is the Audio Mining System from Fraunhofer IAIS, which has been used for several years to explore data collections in ARD or eyewitness interviews in the “German Memory” archive of the Fernuniversität in Hagen.
Despite the impressive capabilities of tools like Audio Mining, new demands and desires for search modalities are emerging from the user side. For example, to find a quote, the exact wording must be largely known so that it can be searched in the transcripts of the automatic speech recognition and directly navigated to the timestamp in a video. However, if the search query is less precise, such as “Find positive/negative statements on topic Y,” more complex search modalities are necessary. The challenge is even greater when search queries include aspects that cannot be answered solely based on the transcript. These can include emotions that are more expressed through facial expressions or a certain tone of voice. A search query like “Statements by person X on topic Y where X was angry or sad” is currently only possible through laborious manual research in the data collections, involving the viewing of many individual videos.
Eyewitness interviews for the exploration of audiovisual cultural heritage
In the project “Multimodal Mining of Eyewitness Interviews for the Exploration of Audiovisual Cultural Heritage,” Fraunhofer IAIS, in cooperation with the Foundation Haus der Geschichte in Bonn, investigates more complex search modalities for a specific application: the exploration of eyewitness interviews.
Through the narratives of eyewitnesses, small and large events in history become vivid and tangible. The medium of audiovisual eyewitness interviews allows these individual and multiperspective narratives to be experienced repeatedly and made accessible to everyone. On www.zeitzeugen-portal.de, there are already over 8,000 clips from about 1,000 eyewitness interviews on German history—a large data collection that not only offers exciting content but also, through emotionally charged stories, allows viewers to empathize with the experiences. An example is our eyewitness Volker Schröder, who participated in student protests in West Berlin in 1968. In his interview, he vividly recounts a situation where he was hit by a police water cannon.
Automated recognition is intended to help us not only focus on what is being told but also how it is being told. Emotions play an important role in the process of remembering, and automated analysis of large data sets can help us better understand the role emotions play in historical recollection.
Multimodal mining
The goal of the project is to develop prototypes for multimodal recognition of perceived emotions and sentiment analyses, which in the medium term will serve as extensions of search modalities for audio mining and be combined with existing analysis algorithms for complex search queries.
The term “recognition of perceived emotions” is intended to highlight how the system is trained and what it can actually do. Unlike the term “emotion recognition” suggests, our system does not aim to truly recognize what a person felt, but rather what other people perceive as emotions when they watch the video of the person (human decoding competence). Thus, the system cannot “read minds,” but only attempts to reproduce the decoding competence of humans. This fine distinction is, in our view, important for precisely and knowledgeably addressing essential ethical and legal questions.
The multimodal recognition is intended to simultaneously combine the video stream (sequence of images), the audio stream, and the transcript (based on automatic speech recognition) to achieve the most accurate recognition possible.
Challenges in applied research
Sentiment analysis and multimodal emotion recognition have been active fields of research for many years, with steadily growing interest. However, the transfer of research results to applications for real-world data poses various challenges.
As with many AI-based approaches, suitable representative training data in adequate scope is one of the main challenges. Many, especially older, research datasets contain acted, often exaggerated emotions that do not nearly reflect the subtly expressed emotions in eyewitness interviews. Datasets with real recordings often show a strong imbalance for certain emotion classes, which often leads to poor learning outcomes for those classes.
Moreover, most datasets are in English. For face-based emotion recognition, this is largely irrelevant—but not for the audio and text modalities. Promising solutions in applied research have been the use of pre-trained models and subsequent adaptation to representative data. The combination of different datasets also seems promising for robust applicability in real applications.
Another major challenge is the human perception of emotions and sentiments themselves. For our analyses, we compiled ten hours of interviews from 147 eyewitnesses and segmented them based on speech pauses. The resulting 2700 segments were annotated by three different people regarding sentiments and emotion classes according to the six typical emotion classes in automatic emotion recognition as per Paul Ekman: joy, sadness, anger, surprise, contempt/disgust, fear. The annotators could assign a score from 0 (neutral) to 3 (strong) for each emotion class and from -3 (very negative) to 3 (very positive) for sentiments. A Spearman correlation analysis between the annotators for each class paints a sobering picture. For sentiments, the correlation is about 0.63. For the emotion class, the value is 0.55, for sadness even only 0.47. For these classes, there is evidently quite a heterogeneous understanding of opinion polarity or perception of emotions among the annotators.
The remaining emotion classes have a correlation of below 0.4, with the emotion class “surprise” having the lowest correlation of only 0.2. For these classes, the annotators very often had a different perception of the displayed emotions.
If even humans have such different perceptions or interpretations of emotions and sentiments, it is to be expected that an AI-based system for such data will hardly be able to achieve precise recognition. Further work in the ongoing research project will show whether and how these limitations can be overcome.
Conclusion
AI-based analyses of audiovisual data can make large data collections and archives searchable for users from various disciplines. The use of AI-based audio processing already allows the search for quotes and spots in videos with certain keywords. For more detailed search queries that go beyond the spoken text, more complex, multimodal analysis algorithms are necessary. These often face significant challenges and limitations in applied research, making it an open and exciting field of applied research.