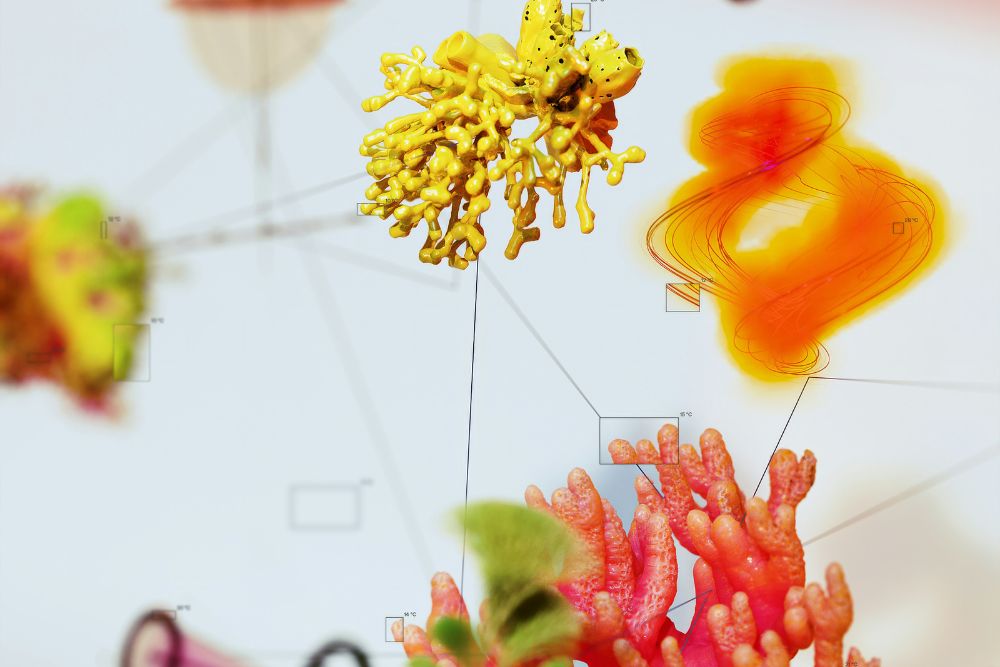
New publication by Lamarr PI Prof. Jürgen Bajorath analyzes the scientific reliability of predictive AI models
The use of artificial intelligence (AI) in the natural sciences continues to grow – particularly in disciplines like chemistry, biology, and medicine. But how meaningful and trustworthy are AI-generated predictions in a scientific context?
This question is addressed in a new publication by Prof. Dr. Jürgen Bajorath, Principal Investigator at the Lamarr Institute for Machine Learning and Artificial Intelligence. He also heads the Life Science Informatics program at the Bonn-Aachen International Center for Information Technology (b-it) at the University of Bonn. His article, From Scientific Theory to Duality of Predictive Artificial Intelligence Models, was recently published in Cell Reports Physical Science.
The study presents a theoretical framework demonstrating that the explanation of AI-generated predictions is not equivalent to their scientific interpretation – and certainly not to the confirmation of causal relationships.
Trustworthy AI in the Natural Sciences: Research at the Lamarr Institute
At the Lamarr Institute, Prof. Bajorath leads the research area Life Sciences. His work combines machine learning methods with applications in cheminformatics, medicinal chemistry, and drug discovery. The new publication addresses one of Lamarr’s central goals: developing explainable and trustworthy AI systems with real-world scientific utility.
In his article, Bajorath introduces the concept of the “duality of predictive models”: Depending on whether a model is based on a sound scientifical rationale s or purely on data patterns, its suitability for follow-up experimentation differs significantly. Only models that are rooted in scientific reasoning, he argues, can reliably support hypothesis generation, exploration of causal relationships , and experimental design.
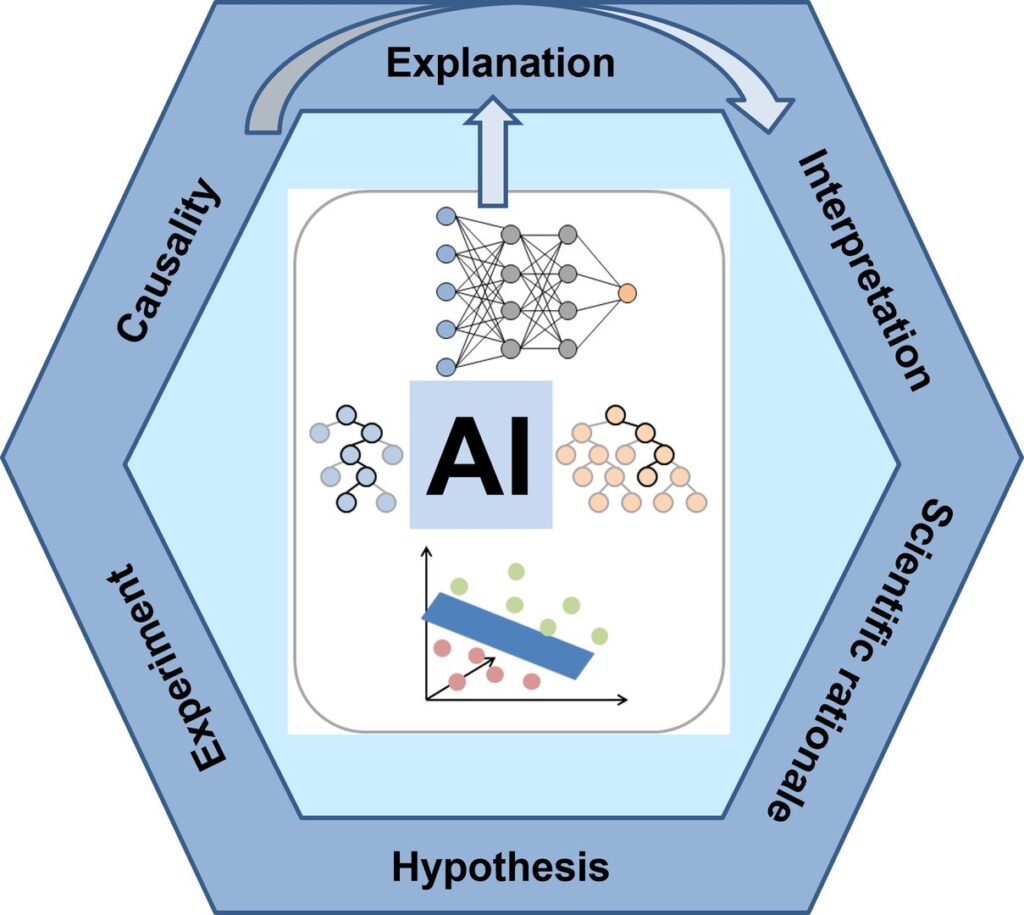
Clear Distinctions: Explanation ≠ Interpretation ≠ Causation
The study systematically distinguishes three key concepts in AI research:
- Explanation: What features does the model use to make its prediction?
- Interpretation: How do humans assess these features in a scientific context?
- Causation: Can a causal relationship be experimentally validated?
When a scientific rationale is lacking as a model’s foundation, misinterpretations are likely – a risk illustrated in the study by so-called “Clever Hans predictors”: models that appear to produce meaningful results but rely on misleading or irrelevant features. This is especially critical in the field of AI in the natural sciences, where correlations are often mistaken for causality.
Relevance for AI Research at the Lamarr Institute
This publication contributes to foundational research in explainable and trustworthy AI, which is one of the Lamarr Institute’s core missions. It clarifies the conditions under which AI in the natural sciences can serve not just as a prediction tool but as a scientifically grounded instrument for hypothesis generation and knowledge discovery.
It also provides valuable input for other Lamarr research areas, such as Trustworthy AI, Hybrid ML, and Life Sciences, which focus on developing explainable and scientifically valid AI models – aligned with the Lamarr Institute’s concept of Triangular AI, which systematically integrates data, knowledge, and context.
Publication: Jürgen Bajorath: From Scientific Theory to Duality of Predictive Artificial Intelligence Models, in: Cell Reports Physical Science (2025) DOI: 10.1016/j.xcrp.2025.102516